1.3. Your First Optimization Problem#
Let’s solve your first optimization problem in Pyomo.
1.3.1. Cloud Computing with Google Colab#
We will include the following code at the top of our notebooks to configure Google Colab.
## Tip: Please put code like this at the top of your notebook.
# We want all of the module/package installations to start up front
import sys
if "google.colab" in sys.modules:
!wget "https://raw.githubusercontent.com/ndcbe/optimization/main/notebooks/helper.py"
import helper
helper.easy_install()
else:
sys.path.insert(0, '../')
import helper
helper.set_plotting_style()
What does this code do? If we run it on Google Colab, the code first downloads helper.py
. This small utility then helps us install Pyomo and the needed solvers (often via IDAES).
1.3.2. Mathematical Model#
Let’s start with a purely mathematical example:
We want to solve the constrained optimization problem numerically.
1.3.3. Define the Model in Pyomo#
Activity
Fill in the missing constraint.
import pyomo.environ as pyo
# Create instance of concrete Pyomo model.
# concrete means all of the sets and model data are specified at the time of model construction.
# In this class, you'll use a concrete model.
m = pyo.ConcreteModel()
## Declare variables with initial values with bounds
m.x1 = pyo.Var(initialize=1, bounds=(-10, 10))
m.x2 = pyo.Var(initialize=1, bounds=(-10, 10))
m.x3 = pyo.Var(initialize=1, bounds=(-10, 10))
## Declare objective
m.OBJ = pyo.Objective(expr=m.x1**2 + 2*m.x2**2 - m.x3, sense = pyo.minimize)
## Declare equality constraints
m.h1 = pyo.Constraint(expr= m.x1 + m.x2 == 1)
# Add your solution here
## Display model
m.pprint()
3 Var Declarations
x1 : Size=1, Index=None
Key : Lower : Value : Upper : Fixed : Stale : Domain
None : -10 : 1 : 10 : False : False : Reals
x2 : Size=1, Index=None
Key : Lower : Value : Upper : Fixed : Stale : Domain
None : -10 : 1 : 10 : False : False : Reals
x3 : Size=1, Index=None
Key : Lower : Value : Upper : Fixed : Stale : Domain
None : -10 : 1 : 10 : False : False : Reals
1 Objective Declarations
OBJ : Size=1, Index=None, Active=True
Key : Active : Sense : Expression
None : True : minimize : x1**2 + 2*x2**2 - x3
2 Constraint Declarations
h1 : Size=1, Index=None, Active=True
Key : Lower : Body : Upper : Active
None : 1.0 : x1 + x2 : 1.0 : True
h2 : Size=1, Index=None, Active=True
Key : Lower : Body : Upper : Active
None : 5.0 : x1 + 2*x2 - x3 : 5.0 : True
6 Declarations: x1 x2 x3 OBJ h1 h2
Click to see the solution to the activity
m.h2 = pyo.Constraint(expr= m.x1 + 2*m.x2 - m.x3 == 5)
1.3.4. Solve using Ipopt#
Toward the end of the semester we will learn, in perhaps more detail than you care, what makes Ipopt work under the hood. For now, we’ll use it as a computational tool.
opt1 = pyo.SolverFactory('ipopt')
status1 = opt1.solve(m, tee=True)
Ipopt 3.13.2:
******************************************************************************
This program contains Ipopt, a library for large-scale nonlinear optimization.
Ipopt is released as open source code under the Eclipse Public License (EPL).
For more information visit http://projects.coin-or.org/Ipopt
This version of Ipopt was compiled from source code available at
https://github.com/IDAES/Ipopt as part of the Institute for the Design of
Advanced Energy Systems Process Systems Engineering Framework (IDAES PSE
Framework) Copyright (c) 2018-2019. See https://github.com/IDAES/idaes-pse.
This version of Ipopt was compiled using HSL, a collection of Fortran codes
for large-scale scientific computation. All technical papers, sales and
publicity material resulting from use of the HSL codes within IPOPT must
contain the following acknowledgement:
HSL, a collection of Fortran codes for large-scale scientific
computation. See http://www.hsl.rl.ac.uk.
******************************************************************************
This is Ipopt version 3.13.2, running with linear solver ma27.
Number of nonzeros in equality constraint Jacobian...: 5
Number of nonzeros in inequality constraint Jacobian.: 0
Number of nonzeros in Lagrangian Hessian.............: 2
Total number of variables............................: 3
variables with only lower bounds: 0
variables with lower and upper bounds: 3
variables with only upper bounds: 0
Total number of equality constraints.................: 2
Total number of inequality constraints...............: 0
inequality constraints with only lower bounds: 0
inequality constraints with lower and upper bounds: 0
inequality constraints with only upper bounds: 0
iter objective inf_pr inf_du lg(mu) ||d|| lg(rg) alpha_du alpha_pr ls
0 2.0000000e+00 3.00e+00 3.33e-01 -1.0 0.00e+00 - 0.00e+00 0.00e+00 0
1 4.3065612e+00 1.11e-16 2.89e-01 -1.0 4.36e+00 - 6.72e-01 1.00e+00h 1
2 4.2501103e+00 0.00e+00 5.55e-17 -1.0 1.31e-01 - 1.00e+00 1.00e+00f 1
3 4.2500000e+00 0.00e+00 4.32e-16 -2.5 6.02e-03 - 1.00e+00 1.00e+00f 1
4 4.2500000e+00 8.88e-16 1.14e-16 -3.8 4.41e-05 - 1.00e+00 1.00e+00f 1
5 4.2500000e+00 0.00e+00 3.13e-16 -5.7 1.98e-06 - 1.00e+00 1.00e+00h 1
6 4.2500000e+00 0.00e+00 1.55e-16 -8.6 2.45e-08 - 1.00e+00 1.00e+00f 1
Number of Iterations....: 6
(scaled) (unscaled)
Objective...............: 4.2500000000000000e+00 4.2500000000000000e+00
Dual infeasibility......: 1.5452628521041094e-16 1.5452628521041094e-16
Constraint violation....: 0.0000000000000000e+00 0.0000000000000000e+00
Complementarity.........: 2.5059105039901454e-09 2.5059105039901454e-09
Overall NLP error.......: 2.5059105039901454e-09 2.5059105039901454e-09
Number of objective function evaluations = 7
Number of objective gradient evaluations = 7
Number of equality constraint evaluations = 7
Number of inequality constraint evaluations = 0
Number of equality constraint Jacobian evaluations = 7
Number of inequality constraint Jacobian evaluations = 0
Number of Lagrangian Hessian evaluations = 6
Total CPU secs in IPOPT (w/o function evaluations) = 0.001
Total CPU secs in NLP function evaluations = 0.000
EXIT: Optimal Solution Found.
1.3.5. Inspect the Solution#
Now let’s inspect the solution. We’ll use the function value()
to extract the numeric value from the Pyomo variable object.
## Return the solution
print("x1 = ",pyo.value(m.x1))
print("x2 = ",pyo.value(m.x2))
print("x3 = ",pyo.value(m.x3))
print("\n")
x1 = 0.4999999999666826
x2 = 0.5000000000333175
x3 = -3.499999999966682
1.3.6. Visualize the Solution#
Is our answer correct?
We can solve this optimization problem with guess and check. If we guess \(x_3\), we can then solve the constraints for \(x_1\) and \(x_2\):
Constraints:
We can then evaluate the objective. Let’s see the graphical solution to our optimization problem.
Activity
Verify you agree with how to translate the two linear constraints into a linear system of questions.
import numpy as np
import matplotlib.pyplot as plt
def constraints(x3):
''' Solve the linear constraints
Args:
x3: Value for the decision variable x3
Returns:
x1 and x2: Values calculated from the constraints
'''
# Define the matrices in the above equations
A = np.array([[1, 1],[1, 2]])
b = np.array([1, 5+x3])
# Solve the linear system of equations
z = np.linalg.solve(A,b)
x1 = z[0]
x2 = z[1]
return x1, x2
# Define a lambda function to plot the objective
objective = lambda x1, x2, x3: x1**2 + 2*x2**2 - x3
# Guess many values of x3.
x3_guesses = np.linspace(-10,4,21)
obj = []
for x3 in x3_guesses:
# Solve the constraints to determine x1 and x2
x1, x2 = constraints(x3)
# Calculate the store the objective function value
obj.append(objective(x1,x2,x3))
# Plot the objective function value versus x3
plt.plot(x3_guesses, obj,color='blue',linewidth=2,label="Sensitivity Analysis")
plt.xlabel("$x_3$",fontsize=18)
plt.ylabel("$f(x)$",fontsize=18)
# Plot the solution from Pyomo
x3_sln = pyo.value(m.x3)
obj_sln = pyo.value(m.OBJ)
plt.plot(x3_sln, obj_sln,marker='o',color='red',markersize=10,label="Pyomo Solution",linestyle='')
plt.legend()
plt.grid(True)
plt.show()
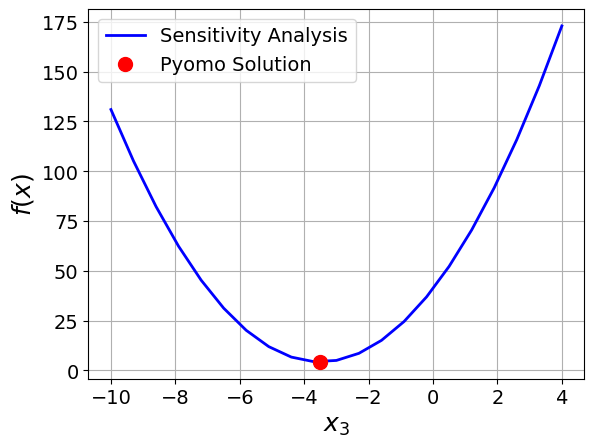